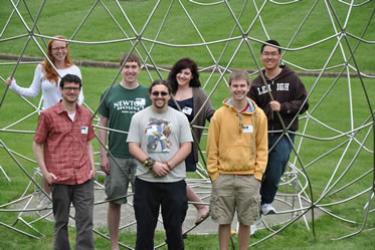
Project Description:
Laboratory experimental evolution, in combination with next generation sequencing technology, is a powerful tool for pursuing a mechanistic understanding of adaptation. For a given selection there are a limited number of accessible pathways to substantially higher fitness. Replicate populations, therefore, tend to find similar adaptive solutions, yet none find the same solution. This is because, fundamentally, adaptation is driven both by the chance effects of mutation and drift and by the deterministic action of selection on a small number of beneficial “driver” mutations.
The objective of this proposal is to exploit an experimental system for identifying biological pathways that regularly yield the mutations that drive adaptation in the yeast Saccharomyces cerevisiae. This work will advance the long-term goal of developing a mechanistic understanding of genome evolution and will provide broad interdisciplinary training in methods spanning molecular biology, evolutionary biology, genetics, genomics, and bioinformatics.
Front row: Gregory Lang, Ph.D., Daniel MMarad, Matthew Messersmith
Back row: Ryan Peace, Thomas Tavolara, Danielle Auth, Kevin Trinh
Project Year:
2014
Team Leaders:
Gregory Lang, Ph.D. (Biological Sciences)
Dan Lopresti, Ph.D. (Computer Science & Engineering)
Graduate Students:
Daniel Marad
Matthew Messersmith
Undergraduate Students:
Danielle Auth
Ryan Peace
Thomas Tavolara
Kevin Trinh